In the fast-paced and ever-evolving world of engineering, where innovation and technological advancements are the driving forces, hiring managers find themselves navigating a challenging landscape.
The role of data science in modern engineer staffing is not just a small piece of the puzzle; it’s a cornerstone that demands our utmost attention. It’s a transformational element that can spell the difference between success and stagnation. By leveraging data-driven insights, hiring managers can make more informed decisions, streamline the recruitment process, and ultimately build and maintain a competitive workforce.
Understanding the Data Science Revolution: A Paradigm Shift in Hiring
Data science isn’t merely a new tool in the toolbox; it’s a fundamental shift in the way we approach engineer staffing. It involves the systematic collection, analysis, and interpretation of data to make highly informed hiring decisions.
Leveraging Big Data: Tapping into the Digital Deluge
We now live in an era of information abundance, where data flows ceaselessly. This flood of digital information, often termed “big data,” can be harnessed to understand market trends, skill demands, and the availability of engineers with unprecedented accuracy.
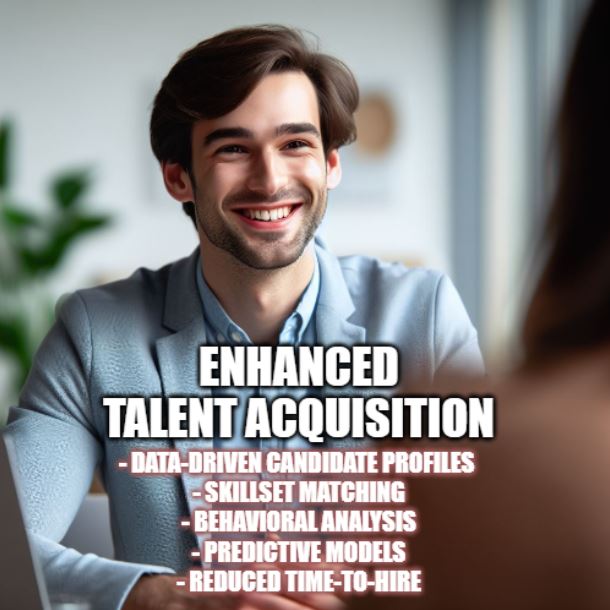
Enhanced Talent Acquisition: A Precision Instrument
Data science isn’t just a magnifying glass; it’s a precision instrument that helps hiring managers identify top engineering talent with surgical accuracy. This capability reduces the risk of poor hiring decisions and enhances your team’s overall competence.
Data-Driven Candidate Profiles
Traditional hiring often involves sifting through stacks of resumes and manually assessing qualifications. With data science, the process becomes much more efficient. By collecting and analyzing data on candidates, including their work history, skills, and even their online presence, engineering staffing agencies and other recruitment entities can create data-driven profiles that provide a holistic view of a candidate’s qualifications and potential cultural fit.
Skillset Matching
In the engineering field, it’s crucial to ensure that the acquired talent possesses the precise skills needed for a particular role. Data science enables the comparison of a candidate’s skillset with the specific requirements of the job. This skillset matching goes beyond keyword matching and dives deep into the nuances of each candidate’s experience, significantly reducing the risk of misalignment between job roles and talent.
Behavioral Analysis
Beyond qualifications, understanding a candidate’s behavior, work habits, and cultural fit is vital for successful talent acquisition. Data science tools can analyze a candidate’s behavior, using data from social media, previous work interactions, and even personality assessments to gauge how well they will integrate into the organization’s culture.
Predictive Models
Data science isn’t just about assessing the current suitability of candidates; it can also predict their future performance and alignment with the organization. Advanced algorithms can use historical data on successful hires to create predictive models that forecast how well a candidate is likely to perform in the future. This predictive analysis is invaluable for organizations looking to make long-term, impactful hires.
Reduced Time-to-Hire
Precision in talent acquisition leads to a quicker hiring process. By swiftly identifying the most suitable candidates, data-driven insights can substantially reduce the time it takes to fill vacant engineering positions. This is particularly critical in a competitive job market where top talent is often snapped up swiftly.
Candidate Experience Enhancement
Data science isn’t just about finding the right candidates; it’s also about making the hiring process smoother for candidates. When the application and interview process is more tailored and efficient, candidates have a better experience, leading to improved relationships with engineering staffing agencies and other recruiting entities.
Predictive Analysis: Peering into the Future
The power of data science extends to predictive analysis. By utilizing predictive models, you can forecast your organization’s future hiring needs and proactively identify potential gaps in your engineering team.
Customizing Job Descriptions: Tailoring Your Needs
Using data-driven insights, you can craft job descriptions that resonate with potential candidates. This personalization enhances the quality and relevance of applications received, saving time and energy for both parties.
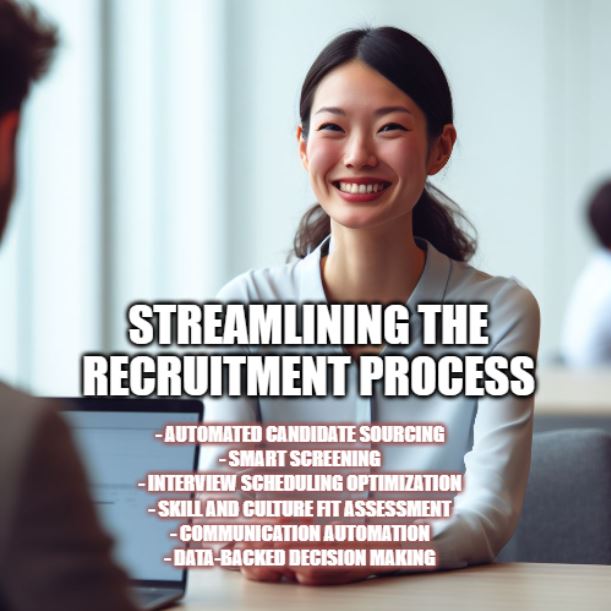
Streamlining the Recruitment Process: Speed and Efficiency
Data-driven insights streamline the recruitment process by making it more efficient. This results in a reduction in time-to-hire, allowing you to onboard talent more quickly and effectively.
Automated Candidate Sourcing
Data science enables the automated sourcing of candidates, significantly reducing the manual effort required. Advanced algorithms can scan through vast databases and online platforms to identify potential candidates who match the job requirements. This not only expedites the process but also ensures that no potential candidate is overlooked.
Smart Screening
Data-driven recruitment processes allow for smart screening, where algorithms evaluate resumes and applications against predetermined criteria. This automated filtering ensures that only the most qualified candidates make it through to the next stages of the hiring process. By eliminating the need for manual review of every application, engineering staffing agencies and other recruitment entities save considerable time and resources.
Interview Scheduling Optimization
Coordinating interviews can be a logistical challenge, especially when dealing with multiple candidates and interviewers. Data science tools can help optimize interview scheduling by considering the availability of both parties and suggesting the most convenient times. This not only reduces scheduling conflicts but also speeds up the interview process.
Skill and Culture Fit Assessment
Efficiency doesn’t mean sacrificing quality. Data science enables the simultaneous assessment of a candidate’s skills and cultural fit. Algorithms can analyze a candidate’s qualifications against the job requirements and, at the same time, evaluate their alignment with the organization’s culture. This dual assessment ensures that candidates are not only technically proficient but also compatible with the company’s values and work environment.
Communication Automation
Effective communication with candidates is crucial for maintaining a positive candidate experience. Data science allows for automated communication, including status updates, interview invitations, and feedback sharing. Automated communications keep candidates informed and engaged throughout the process, all while freeing up recruiters’ time.
Reducing Time-to-Hire
One of the most significant advantages of streamlining the recruitment process is the reduction in time-to-hire. Data-driven processes, by eliminating bottlenecks and inefficiencies, help engineering staffing firms and other recruitment entities fill positions faster. This is particularly vital in a competitive job market where quick hiring decisions can make the difference between securing top talent and losing out to competitors.
Data-Backed Decision Making
Streamlining doesn’t mean simplifying to the point of oversight. Data science provides decision-makers with real-time insights into the recruitment process. Through dashboards and analytics, hiring managers and engineering staffing agencies can stay informed about the progress and make informed adjustments as necessary. This ability to make data-backed decisions contributes to efficiency by preventing mistakes and misalignment in the recruitment process.
Skill Gap Analysis: Bridging the Gaps
Data science can identify gaps in your engineering team’s skills. Armed with this knowledge, you can prioritize skill development programs and ensure your team’s proficiency remains up-to-date.
Compensation Analysis: Attracting the Best
To secure the best engineering talent, it’s crucial that your compensation packages are not only competitive but also optimized. Data science provides the means to set your offerings at the sweet spot.
Candidate Assessment: Evaluating Potential Stars
Leveraging data, you can design highly effective assessment methods for potential hires, ensuring you identify the best fit for your organization.
Reducing Bias in Hiring: Promoting Inclusivity
Data-driven decision-making is a powerful weapon against unconscious biases. It promotes diversity and inclusion by reducing human prejudice in the hiring process.
Building Talent Pipelines: A Continuous Flow
Data science also aids in identifying and nurturing potential candidates. By building talent pipelines, you ensure a steady flow of top talent when needed, reducing the strain of last-minute hirings.
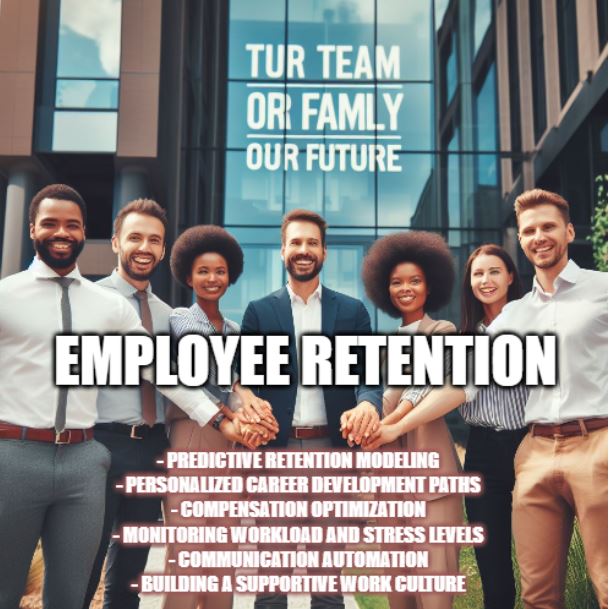
Employee Retention: A Crucial Aspect
Employee retention is as important as recruitment. Data science can identify factors contributing to turnover, helping you create a work environment that engineers want to stay in.
Identifying Key Retention Factors
Data science allows organizations to analyze a plethora of data points to identify the key factors contributing to employee turnover. These factors could range from job satisfaction, work-life balance, career development opportunities, compensation, or even the relationships between team members. By pinpointing these factors, organizations can address issues that are leading to attrition more precisely.
Predictive Retention Modeling
Advanced predictive models can forecast which employees are more likely to leave the organization in the future. These models take into account historical data, such as turnover rates, as well as various factors related to an employee’s performance and engagement. With this information, engineering staffing agencies and other recruitment entities can proactively take steps to retain valuable employees before they decide to leave.
Personalized Career Development Paths
Data-driven insights can help tailor career development paths for individual engineers within an organization. By analyzing their skills, experiences, and career aspirations, organizations can provide personalized development opportunities, ensuring that employees feel they are growing in their roles. This personalization boosts job satisfaction and, in turn, retention.
Compensation Optimization
Data science enables organizations to optimize their compensation packages, ensuring that they are competitive and attractive to engineering talent. By analyzing market data, organizations can ensure that their pay scales are in line with industry standards, reducing the temptation for engineers to leave for higher-paying positions elsewhere.
Employee Engagement Analysis
Employee engagement is closely tied to retention. Data science tools can measure and evaluate employee engagement through surveys, feedback, and other metrics. This analysis provides insights into how engaged employees are and what measures can be taken to improve their experience within the organization.
Monitoring Workload and Stress Levels
The engineering field often comes with high-pressure work environments. Data science can help in monitoring workload and stress levels among employees. By analyzing these data points, organizations can intervene proactively to alleviate excessive stress and prevent employee burnout, a major contributor to attrition.
Building a Supportive Work Culture
Data can also be used to analyze the dynamics within teams and the broader organization culture. It identifies areas that need improvement to create a more supportive work environment. Employees who feel supported and valued are more likely to stay with the company.
Maximizing ROI: Every Hire Counts
It’s not just about hiring; it’s about ensuring that every hire contributes positively to your organization’s success. Data-driven decisions help you maximize the return on investment in your talent.
Real-time Market Insights: Staying Ahead
With data science, you can stay updated with real-time market trends. This allows you to adapt your staffing strategies proactively, ensuring you’re always ahead of the curve.
Monitoring Staffing Costs: Efficient Budgeting
Data science isn’t just about hiring; it’s also about managing staffing costs efficiently and optimizing your budget to get the best value for your investment.
Scaling for Growth: Adapting to Change
As organizations grow, they need to scale their staffing strategies. Data science assists in creating scalable staffing strategies that adapt to your organization’s growth, maintaining a competitive edge even as your team expands.
Data science is not merely an optional tool in modern engineer staffing; it is an indispensable pillar. The ability to gather, analyze, and apply data-driven insights to the hiring process is essential for maintaining a competitive edge in the engineering industry.
By embracing the data science revolution, hiring managers can make smarter decisions, reduce bias, and ensure their teams are well-equipped to meet the demands of a rapidly evolving technological landscape. Embrace the data science revolution in engineer staffing and unlock the full potential of your recruitment efforts. Your future success depends on it.
Data Science Information
- High Demand: Data science is one of the most in-demand fields, with an ever-growing need for professionals who can work with data to derive insights. It consistently ranks as one of the top job fields. Source: Glassdoor
- Salary Growth: Data science roles offer competitive salaries and high earning potential. Data scientists often command some of the best-paying jobs in the market. Source: PayScale
- Data Science Community: Data science has a vibrant and active online community where professionals share knowledge, resources, and collaborate on projects. Platforms like Kaggle and Stack Overflow are valuable resources. Source: Kaggle and Stack Overflow