Data Scientist Staffing Firm
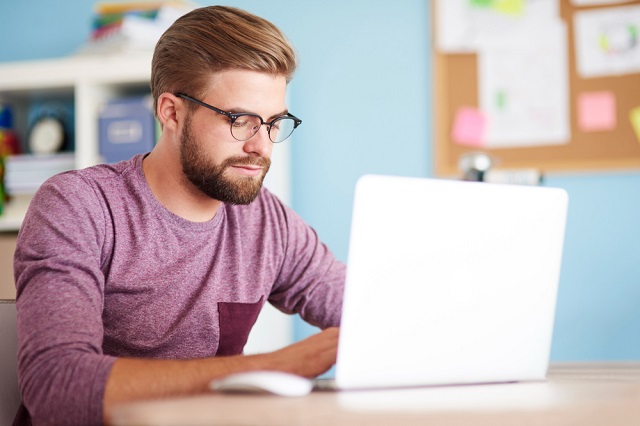
Find Top Talent in Your Area
Tier2Tek Recruitment Agency is the premier choice for companies looking to hire Data Scientists. Our expertise in staffing, recruitment, and direct hire placement ensures that your organization gets the top talent it needs.
Why Choose Tier2Tek for Your Team
Selecting the right recruitment partner is crucial. Here are compelling reasons to choose Tier2Tek Staffing:
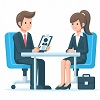
Sourcing Speed
Our recruitment agency excels in quickly identifying and presenting top Data Scientist candidates to meet your urgent needs.
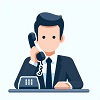
Communication
We maintain clear and consistent communication throughout the hiring process to ensure alignment with your requirements for a Data Scientist.
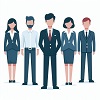
Quality Candidates
Our placement agency focuses on sourcing highly qualified candidates to ensure that you hire the best Data Scientists available.
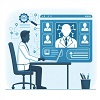
Innovative Sourcing
Utilizing cutting-edge sourcing methods, our employment agency ensures we find the most suitable Data Scientist talent for your organization.
Our Impressive Statistics
98%
High Placement Success Rate
Our agency’s exceptional placement rate ensures your company will hire the right Data Scientist.
☝ Week
Rapid Placement Times
We pride ourselves on our ability to place candidates swiftly, with most positions filled within a week.
95%
Client Retention
Our high client retention rate speaks volumes about the satisfaction of hiring managers with our services.
Testimonials from Hiring Managers
HEAD OF ANALYTICS
“Tier2Tek helped us hire a skilled Data Scientist in record time. Their understanding of our needs was unparalleled.”
CTO
“The recruitment agency provided us with top-tier Data Scientist candidates who fit perfectly into our team. Their process is seamless.”
HR DIRECTOR
“The quality and speed of Tier2Tek’s service were impressive. We quickly found a Data Scientist who exceeded our expectations.”
Our Streamlined Hiring Process
✓ Understanding Your Unique Needs: Every company is different; we tailor our approach to fit your organization’s culture and goals.
✓ Start Sourcing: We begin sourcing candidates using innovative strategies to find the best talent.
✓ Interviewing: We conduct thorough interviews to assess candidates’ fit for your Data Scientist position.
✓ Submitting Only the Best: Only the top candidates are presented to you for consideration.
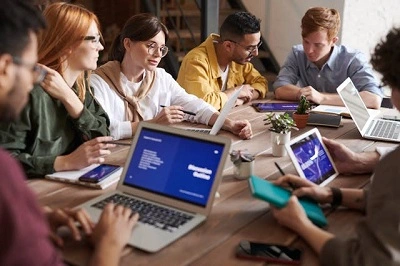
Comprehensive Candidate Screening
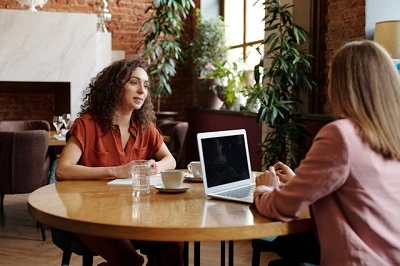
✓ Professionalism: We look for candidates who demonstrate a high level of professionalism suitable for your Data Scientist role.
✓ Communication Skills: Effective communication is crucial; we ensure candidates can clearly convey complex ideas.
✓ Technical Aptitude: Candidates must have strong technical skills to meet the demands of a Data Scientist position.
✓ Strong Work History: We prioritize candidates with proven track records of success in their previous roles.
Respect for Privacy
We respect the privacy of both our clients and candidates, ensuring confidentiality throughout the hiring process.
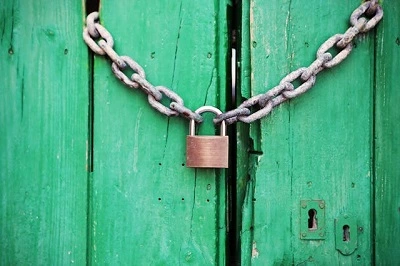
Our Guarantee
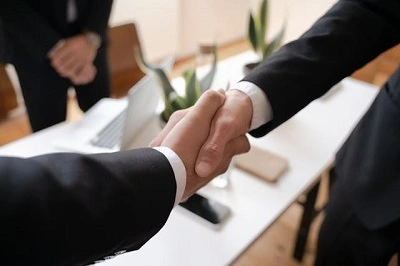
If a candidate does not work out, we are committed to finding a replacement promptly. Your satisfaction is our top priority.
By choosing Tier2Tek Recruitment Agency, you ensure your company hires the most qualified Data Scientist professionals efficiently and effectively.
Sample Job Description for Data Scientist
About the Role: The Data Scientist is a pivotal team member responsible for leveraging data to derive actionable insights and drive business decisions. This role involves analyzing large data sets, developing statistical models, and implementing machine learning algorithms. The Data Scientist will collaborate with cross-functional teams to understand data requirements, provide analytical support, and communicate findings effectively.
Key Responsibilities:
- Develop and implement advanced data analysis, predictive analytics, and machine learning models.
- Perform statistical analysis to identify trends, patterns, and insights from complex data sets.
- Collaborate with stakeholders to define data requirements and objectives.
- Clean, preprocess, and verify the integrity of data used for analysis.
- Present findings and recommendations to stakeholders in a clear and concise manner.
- Stay abreast of industry trends and advancements in data science and analytics.
Required Skills and Qualifications:
- Bachelor’s or Master’s degree in Computer Science, Statistics, Mathematics, or a related field.
- Proven experience in data science, analytics, or a related role.
- Strong proficiency in programming languages such as Python, R, or SQL.
- Experience with machine learning techniques and algorithms.
- Familiarity with data visualization tools and techniques.
- Excellent analytical and problem-solving skills.
- Strong communication and collaboration abilities.
Preferred Experience:
- Experience with big data technologies such as Hadoop, Spark, or similar.
- Knowledge of cloud computing platforms like AWS, Azure, or Google Cloud.
- Experience in a specific industry sector (e.g., finance, healthcare, retail) is a plus.
Common Technologies That a Data Scientist Uses
Data Scientists commonly use a variety of technologies and tools to perform their tasks, which include data analysis, machine learning, statistical modeling, and data visualization. Some of the key technologies used by Data Scientists are:
- Programming Languages:
- Python: Widely used due to its simplicity and the vast array of data science libraries available, such as Pandas, NumPy, Scikit-learn, TensorFlow, and Keras.
- R: Popular for statistical analysis and visualization, with packages like ggplot2, dplyr, and shiny.
- Big Data Technologies:
- Apache Hadoop: A framework for distributed storage and processing of big data using the MapReduce programming model.
- Apache Spark: An engine for large-scale data processing that is faster than Hadoop for certain applications.
- Databases and Data Warehousing:
- SQL: Essential for data retrieval and manipulation in relational databases.
- NoSQL databases (e.g., MongoDB, Cassandra): Used for working with unstructured data or when scalability and performance are key.
- Data warehousing solutions like Amazon Redshift, Google BigQuery, or Snowflake.
- Machine Learning and Deep Learning Frameworks:
- TensorFlow and Keras: Popular for building neural networks and deep learning models.
- PyTorch: Another deep learning framework known for its flexibility and ease of use.
- Data Visualization Tools:
- Tableau: Widely used for creating interactive and shareable dashboards.
- Power BI: A Microsoft tool for data visualization and business analytics.
- Matplotlib and Seaborn: Python libraries for creating static, animated, and interactive visualizations.
- Cloud Computing Platforms:
- AWS (Amazon Web Services): Offers various services like EC2, S3, and AWS Lambda.
- Microsoft Azure: Provides a range of cloud services including Azure Machine Learning.
- Google Cloud Platform: Known for services like Google Colab and BigQuery.
- Version Control Systems:
- Git: Essential for version control, often used with platforms like GitHub or GitLab for collaboration and code management.
- Data Integration Tools:
- Apache NiFi or Talend: For data flow automation and management.
- Business Intelligence Tools:
- QlikView or SAS Business Intelligence for more traditional BI tasks.
Data Scientists may use a combination of these technologies depending on the specific needs of their projects and the industry they are working in.
Common Expertise of Data Scientists
- Statistical Analysis: Applying statistical methods to extract insights from complex datasets.
- Machine Learning: Building predictive models using supervised and unsupervised learning techniques.
- Data Visualization: Presenting data insights through clear and compelling visual representations.
- Programming: Utilizing programming languages like Python, R, and SQL to manipulate and analyze data.
- Big Data Technologies: Working with Hadoop, Spark, and similar tools to manage large-scale data processing.
- Domain Knowledge: Understanding specific industry nuances to deliver actionable business insights.